Forecasting Methods Compare and Contrast
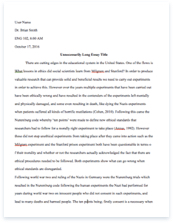
- Pages: 6
- Word count: 1278
- Category: Contrast Forecasting Future
A limited time offer! Get a custom sample essay written according to your requirements urgent 3h delivery guaranteed
Order NowForecast in a simple terms is a prediction thru a statement or claim that a particular event will occur in the future. It looks like to me that almost the majority of the people, including children, once in their life time were a forecasters, as sometimes in their past theyve tried to predict any future event. This act of making such prediction is therefore, called forecasting. Forecasts are never finished, they are needed continuously and as the time passes, their accuracy and their impact on actual performance are meas So it looks like that forecast in itself, is not too complicated, it becomes complicated once the word good is attached to it.
Thus, the forecast has to be well thought and planned so it can be called good or adequate forecasting. In order to prepare a forecast, one should first identify the assumptions in the forecast model one intent to use. Then gather and analyze relevant data. After that, forecast and finally, monitor the results to whether or not the trend has weakened, strengthened, or if there is any factors that have gone unchanged. Forecast should be timely, accurate, reliable, and the technique should be simple to understand and yet, cost-effective (McGraw-Hill, 2005).
Obtaining a reliable and accurate forecasting of future sales or market behavior is of paramount importance for business people such as policy makers, companies making investment decisions, setting up prices and so on. There are several forecasting methods and they are differently used by different companies to satisfy each one specific needs. Forecasting models companies use depend mainly of factors such as accuracy required, time horizon, availability of data and competent personnel (1995, Colgate, et al).
Forecast can be long-term or short-term. The techniques used in forecasting may be grouped into two categories: qualitative or quantitative. Qualitative is very subjective and it consists primarily of personal opinions or inputs quite difficult or impossible to quantify. Consumer survey (sample of consumer opinions), sales based on contact with customers and market research are clear examples of qualitative forecast methods. The disadvantages of this model is that it requires considerable amount of time, skills and knowledge to put together a good survey, manage it and interpret the results for valid use. On the other hand, quantitative forecasting consists primarily in analyzing objective data and they may be subdivided into two categories: 1) Time series, where future values are estimated from past values of the series and 2) casual models, which normally uses the linear regression technique (McGraw-Hill 2005). Quantitative forecasting tends to circumvent personal biases, which are often times the source of contamination on qualitative method.
Time series averaging technique, which is based on past data, is a very simple technique that has basically no cost, quick and easy to prepare and yet, easy to understand.
Among all the forecasting models, exponential smoothing is the most used of all forecasting techniques(Operations Management, University of Phoenix Custom edition e-test 2005) as it is almost found in every computerized forecasting software. Its popularity is due to the fact that it is the most reliable among all other models, easy to formulate and compute, and finally its easiness to check its accuracy.
Every organization needs forecasts for better business operations, and they should be produced by a group of forecasters, not individually. In general, the decision-makers use forecasting models to assist them in their decision-making process. The figure bellow depicts the model overview of business forecasting techniques.
With forecast being an uncertain process of prediction of what will occur in the future, then, because of this uncertainty, the accuracy of a forecast is as important as the outcome predicted by the forecast itself. One sector of the economy that lately needs a very accurate forecast is the airlines industry. With the event of September 11, 2001, this industry got hit very hard as some companies have already declared bankruptcy. Delta airline is one clear example as it is the latest one to make use of the chapter 11. The remaining of this paper will be talking about the different forecasting methods used by the airline industry to make future prediction of passenger demand. The exactness of forecasts is very critical to the revenue management system as lousy prediction of demand can lead to an incorrect decision-making, which in turn leads to a miserable revenue performance. So the airline industry, more than any other industry, needs an accurate forecasting not only because of the September 11 occurrence, but also due to a very high price of crude oil, which tends to increase the price of kerosene highly used by the industry.
One study identified as the Improved Forecast Accuracy in Airline Revenue Management (2001, Richard Zeni) shows that airlines divide passengers into two large categories based on their sensitivity to price and travel needs. These included business passengers and leisure passengers. This study has further shown that business travelers have a tendency to make their arrangements close to their departure date staying at their destination for only a short time. However, they did not show much flexibility on their plans at all, but they were willingly to pay more money for their tickets. On the other hand, exactly the opposite happened with leisure travelers. They usually booked their flights well ahead of their travel date; stayed more time at their final destinations, were more flexible by even refusing to travel rather then paying higher tickets prices. Such behavior is usually costly to the airlines because planes sometimes take off with empty seats. Therefore, I believe this aspect is very crucial to the airline forecasting and it serves a stepping-stone to good airline forecasting.
The challenge to the airline decision-makers then becomes: how to make these empty seats available at lower price and at the same time, preventing those travelers eagerly to pay higher prices from getting their hand on these tickets. Therefore, forecasters must forecast how many low-fare ticket buyers (leisure travelers) are willing to book a flight so to protect just enough seats to the business passengers as they require them. Airline industry usually uses historical data to forecast their passengers traveling needs although these data do not reveal the true demand. If censored data is used as their historical demand, this tend to result in too few seats saved for high-fare passengers, which means that seats that could be sold to high-fare travelers are sold to low-fare travelers and this translates into a negative revenue. So, it is in the airline interests to change censored data into more factual historical data.
Airlines usually overbook flights based on the expectation that some passengers will not show. An accurate forecast number of these no show travelers is said to increase airline revenue by reducing the numbers of unused seats (Lawrence, et al 2004). A study entitled passenger based predictive modeling of airline used two models of forecasting to predict the no-show rates.
Airlines make use of censored data to determine their forecast of demand and revenue management system to calculate how many seats must be reserved at different prices.
References
Bureau of Transportation Statistics. (2005). Air traffic statistics and airline financial statistics. Retrieved February 17, 2006 from http://www.bts.gov/oai/indicators JetBlue Airways Corporation. (2002). Jetblues 2002 Form 10-K. Retrieved February 22, 2006, from http://media.corporate-ir.net/media_files.
Improved Forecast Accuracy in Airline Revenue Management by Unconstraining Demand estimates from censored Data. Retrieved February 20, 2006 from http://www.bookpump.com/aps/pdfb University of Phoenix. (Ed.) (2005). Statistical Techniques in Business & Economics, 12th Edition [University of Phoenix Custom Edition e-text]. The McGraw-Hill Co. Last retrieved on February 20th, 2006 from University of Phoenix student Resource site MGT 554