Data Mining
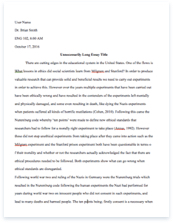
- Pages: 3
- Word count: 717
- Category: College Example
A limited time offer! Get a custom sample essay written according to your requirements urgent 3h delivery guaranteed
Order NowSimply stated data mining refers to extracting or mining knowledge from large amounts of it. The term is actually a misnomer. Remember that the mining of gold from rocks or sand is referred to as gold mining rather than rock or sand mining. Thus, data mining should have been more appropriately named “knowledge mining from data,” which is unfortunately somewhat long. Knowledge mining, a shorter term, may not re? ect the emphasis on mining from large amounts of data.
Nevertheless, mining is a vivid term characterizing the process that ? nds a small set of precious nuggets from a great deal of raw material Thus, such a misnomer that carries both “data” and mining became a popular choice. Many other terms carry a similar or slightly different meaning to data mining, such as knowledge mining from data, knowledge extraction, data pattern analysis, data archaeology, and data dredging The definition above refers to observational data, as opposed to experimental data.
Data mining typically deals with data that have already been collected for some purpose other than the data mining analysis (for example, they may have been collected in order to maintain an up-to-date record of all the transactions in a bank). This means that the objectives of the data mining exercise play no role in the data collection strategy. This is one way in which data mining differs from much of statistics, in which data are often collected by using efficient strategies to answer specific questions. For this reason, data mining is often referred to as secondary data analysis.
The definition also mentions that the data sets examined in data mining are often large. If only small data sets were involved, we would merely discussing classical exploratory data analysis as practiced by statisticians. When we are faced with large bodies of data, new problems arise. Some of these relate to housekeeping issues of how to store or access the data, but others relate to more fundamental issues, such as how to determine the representativeness of the data, how to analyze the data in a reasonable period of time, and how to decide whether an apparent relationship is merely a chance occurrence not reflecting any underlying reality.
Often the available data comprise only a sample from the complete population or perhaps from a hypothetical super population the aim may be to generalize from the sample to the population. For example, we might wish to predict how future customers are likely to behave or to determine the properties of protein structures that we have not yet seen. Such generalizations may not be achievable through standard statistical approaches because often the data are not classical statistical “random samples, but rather convenience or opportunity samples.
Sometimes we may want to summarize or compress a very large data set in such a way that the result is more comprehensible, without any notion of generalization. This issue would arise, for example, if we had complete census data for a particular country or a database recording millions of individual retail transactions. Although data mining is a relatively young field with many issues that still need to be researched in depth, many off-the-shelf data mining system products and domain speci? c data mining application software’s are available.
As a discipline, data mining has a relatively short history and is constantly evolving new data mining systems appear on the market every year; new functions, features, and visualization tools are added to existing systems on a constant basis; and efforts toward the standardization of data mining language are still underway. Therefore, it is not our intention in this book to provide a detailed description of commercial data mining systems. Instead, we describe the features to consider when selecting a data mining product and offer a quick introduction to a few typical data mining systems.
Reference articles, websites, and recent surveys of Data mining systems are listed in the bibliographic notes. t data description alone cannot provide an action plan. You must build a predictive model based. Patrice:: I thought you did a nice job explaining all of your points, also, see many illustrations in your structures. I got the idea of your paper ;: I really like how the introduction leads into the thesis, explaining the topic in details . I really like your work in data mining at all good luck my classmates .