Regression and Correlation Analysis
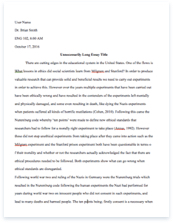
- Pages: 5
- Word count: 1105
- Category: College Example
A limited time offer! Get a custom sample essay written according to your requirements urgent 3h delivery guaranteed
Order Now1. Generate a scatterplot for income ($1,000) versus credit balance ($), including the graph of the best fit line. Interpret.
This scatter plot graph is a representation of combining income and credit balance. It shows the income increasing as the credit balance increases. As a result of this data it can be inferred that there is a positive relationship between the two variables. Because of the positive relationship between income and credit balance the best fit line or linear regression line fits the data quite well. The speculation can be strongly made that the customer with the largest income will, more than likely, have the largest credit balance.
2. Determine the equation of the best fit line, which describes the relationship between income and credit balance.
Regression Analysis: Income($1000) versus Credit Balance($)
The regression equation is
Income($1000) = – 3.52 + 0.0119 Credit Balance($)
Predictor Coef SE Coef T P
Constant -3.516 5.483 -0.64 0.524
Credit Balance($) 0.011926 0.001289 9.25 0.000
S = 8.40667 R-Sq = 64.1% R-Sq(adj) = 63.3%
Analysis of Variance
Source DF SS MS F P
Regression 1 6052.7 6052.7 85.65 0.000
Residual Error 48 3392.3 70.7
Total 49 9445.0
This MiniTab output shows the equation of the best fit line in the income. Income = – 3.52 + 0.0119 Credit Balance ($)
The credit balance is represented by the $ and the income is represented by the $1000s.
3. Determine the coefficient of correlation. Interpret. The coefficient of correlation is 0.801. The positive value of the correlation coefficient shows that there is a strong positive correlation with the two variables. If one of them goes up or down, the other variable will match that move up or down; more than likely by the same unit.
4. Determine the coefficient of determination. Interpret. The coefficient of determination is 0.641. This shows the strength of the prediction of the dependent variable in relation to the independent variable. 0.641 predicts the income is explained by the regression model. The moderate value is inferring that the model would be a medium fit.
5. Test the utility of this regression model (use a two tail test with α =.05). Interpret your results, including the p-value. Using a t-test to test β1 showed the test statistic to be 9.25 in conjunction with the p-value of 0.000. Because the p-value is smaller than a= 0.05, the model is significant.
6. Based on your findings in 1–5, what is your opinion about using credit balance to predict income? Explain My opinion of the model is that it is significant. This mean the independent variable is significant when trying to anticipate the dependent variable. Basically, credit balance can predict income at a fairly high success rate.
7. Compute the 95% confidence interval for beta-1 (the population slope). Interpret this interval. The confidence interval for 95% of β1 is (0.009, 0.015). This suggests the true value of the β1 is within the parameter with 95% surety.
8. Using an interval, estimate the average income for customers that have credit balance of $4,000. Interpret this interval. The average income for customer’s interval is (41.77, 46.61). This shows the data of this interval has a new estimated income for a customer with a 0.95 probability on those who have a credit balance of $4000.
9. Using an interval, predict the income for a customer that has a credit balance of $4,000. Interpret this interval. The interval of (27.11, 61.27) predicts a new income for a customer having a $4000 credit balance with to be within this with a 0.95 probability rate.
10. What can we say about the income for a customer that has a credit balance of $10,000? Explain your answer.
With the credit balance at $10,000 put into the regression model, it shows;
Income = -3.516+ 0.011926*10,000 = 115.7482703.
A customer with a $10,000 credit balance is, more than likely, going to have an income of $115,748.27. That is according to the fitted regression model.
In an attempt to improve the model, we attempt to do a multiple regression model predicting income based on credit balance, years, and size.
11. Using MINITAB, run the multiple regression analysis using the variables credit balance, years, and size to predict income. State the equation for this multiple regression model.
Regression Analysis: Income($1000) versus Credit Balance($), Size, Years
The regression equation is
Income($1000) = – 13.2 + 0.0108 Credit Balance($) + 0.615 Size + 1.21 Years
Predictor Coef SE Coef T P
Constant -13.186 3.608 -3.65 0.001
Credit Balance($) 0.0107922 0.0008184 13.19 0.000
Size 0.6151 0.4178 1.47 0.148
Years 1.2097 0.2322 5.21 0.000
S = 5.26121 R-Sq = 86.5% R-Sq(adj) = 85.6%
Analysis of Variance
Source DF SS MS F P
Regression 3 8171.7 2723.9 98.41 0.000
Residual Error 46 1273.3 27.7
Total 49 9445.0
Source DF Seq SS
Credit Balance($) 1 6052.7
Size 1 1368.0
Years 1 750.9
The fitted regression line:
Income = -13.186 +0.0107922* Credit Balance + 0.6151* Size + 1.2097*Years.
12. Perform the global test foruUtility (F-Test). Explain your conclusion. The F-test shows the statistic in this case to be 98.41 with an accompanying p-value of 0. The null hypothesis will be rejected as a result and will establish the regression model to be significant in predicting the variable of income.
13. Perform the t-test on each independent variable. Explain your conclusions and clearly state how you should proceed. In particular, state which independent variables should we keep and which should be discarded. The t-test statistic of credit balance is 13.19 with the accompanying p-value of 0. In regards to size, it is 1.47 with the accompanying p-value of 0.148. For years it is 5.21 with the p-value of 0. Both credit balance and years for smaller than a=0.05, as a result it shows they are both significant to the prediction of income and should stay in the model. The p-value for size, however, is larger than a=0.05, so it should be removed from the model.
14. Is this multiple regression model better than the linear model that we generated in parts 1–10? Explain.
I believe the multiple regression model is significantly better than the linear model to determine the prediction of credit balance and income variances. This is due to the fact the coefficient of determination, present in both models, has a higher percentage of probability (86.5% vs. 64.1%) in the multiple regression model that the linear. The more variables, the more accurate the inferences, I believe. AJ Davis would be best served by utilizing a multiple regression model and basing the future business and marketing strategies based off all this information.