The Mathematics Modeling of Disease and Vaccination
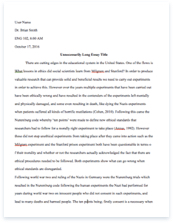
- Pages: 10
- Word count: 2401
- Category: Illness Medicine Vaccination
A limited time offer! Get a custom sample essay written according to your requirements urgent 3h delivery guaranteed
Order NowFrom time immemorial, diseases have been a source of fear and superstition. Throughout history we have seen pandemic diseases such as the Black Death in Middle Ages Europe and the Spanish Flu at the beginning of the 20th century (Scientific American, 2008). More recently we have seen HIV responsible for millions of deaths. In the last few years there have been scares over bird flu, SARS and Ebola â yet none fully developed into a major global health problem. Many, such as the common cold, are minor and are purely an annoyance; but others, such as Ebola or AIDS, fill us with dread. It is the seemingly unpredictable nature of diseases, with some people getting infected while others escape that has gripped our imagination. However, diseases are an inescapable part of human life and to alleviate some of the uncertainty associated with getting infected, mathematics can be used to understand and predict the spread of diseases, relating important publicâhealth questions to the basic parameters of infection (Keeling, 2001). Additionally, vaccination which is a powerful tool in the disease control arsenal, allows for the mass prevention of infection rather than treating the symptoms of infection, thus allowing a higher level of prediction regarding the spread of diseases (Scherer and MacLean, 2002). In this paper, I shall focus on the simplest of disease models and consider some of the more mathematical developments that have improved our predictive ability of the spread of disease by focusing on the use of mathematics in public health and to understand the impact of vaccination at the population level.
MATHEMATICAL MODELING OF INFECTIOUS DISEASES: The mathematics of disease is a dataâdriven subject. Although some purely theoretical work can be done for predictive purposes, the main thrust is to link mathematical models and data. Reports of disease cases from physicians provide us with one of the most detailed sources of biological data; including the number of weekly disease cases for a variety of communities over many years. The signature of social effects, such as changes in birth rate or the increased social interactions during school terms overlies this data (IB Math Resources, May 2014). Therefore, a comprehensive picture of disease dynamics requires a variety of mathematical tools, including creation of a mathematical model leading to differential equations and statistical analysis.
HOW CAN MATHEMATICAL MODELS HELP? Models aid in the understanding of the transmission of infections in a population, to interpret observed epidemiological trends, to identify key determinants of epidemics, to guide the collection of data, to forecast the future direction of an epidemic and to evaluate the potential impact of an intervention (Keeling, 2001). Using mathematics to model the spread of diseases is one of the key steps of preparing for potential new outbreaks of disease. Besides providing information to health workers about the levels of vaccination needed to protect a population, it also helps govern first response actions when new diseases potentially emerge on a global scale (for example, Bird flu, SARS and Ebola have all merited much study over the past few years).
SIR MATHEMATICAL MODEL OF DISEASE : (Smith and Moore, 2004) Almost all mathematical models of diseases start from the same basic premise: that the population can be subdivided into a set of distinct classes, dependent upon their experience with respect to the disease. The most basic of these models classifies individuals as one of susceptible, infectious or recovered. This is termed the SIR model (represented by the picture above, Fig 1). As the first step in the modeling process, we identify the independent and dependent variables. The independent variable is time t, measured in days. We consider two related sets of dependent variables: The first set of depen[image: ]dent variables counts people in each of the groups, each as a function of time: The second set of dependent variables represents the fraction of the total population in each of the three [image: ]categories. So, if N is the total population, we have fractions of population as represented in Table 2. The SIR model looks at how much of the population is susceptible to infection, how many of these go on to become infectious, and how many of these go on to recover (and in what timeframe). There are several assumptions in this model. The population is fixed, no entries (births) or exits (deaths). Individuals are born into the susceptible class. Susceptible individuals have never come into contact with the disease and are able to catch the disease, after which they move into the infectious class. Infectious individuals spread the disease to the susceptibles, and remain in the infectious class for a given period of time (the infectious period) before moving into the recovered class. Finally, individuals in the recovered class are assumed to be immune for life. Fig. 1: MOVEMENT RATES BETWEEN SIR CLASSES
DIFFERENTIAL EQUATIONS IN REAL LIFE : We can make this description more mathematical by formulating a differential equation for the proportion of individuals in each class. Differential equations have a remarkable ability to predict the world around us (IB Maths Resources, Feb 2014). They are used in a wide variety of disciplines, from biology, economics, physics, chemistry and engineering. They can describe exponential growth and decay, the population growth of species or the change in investment return over time. A differential equation is a mathematical equation that involves variables like x or y, as well as the rate at which those variables change. Differential equations are special because the solution of a differential equation is itself a function instead of a number. A differential equation is one which is written in the form dy/dx = âŚâŚâŚ.Some of these can be solved (to get y = âŚ..) simply by integrating, while others require much more complex mathematics. Some differential equations can be solved exactly, and some cannot. Sometimes the solutions can only be estimated, and a computer program is exploited to do this rapidly.
DIFFERENTIAL EQUATIONS AND APPLICATIONS TO MODELLING: (Smith and Moore, 2004) The pool of susceptible individuals will decline over the course of the epidemic as new individuals become infected. Without births or immigration, there is no way to generate additional susceptible individuals in this model. If we assume random encounters [image: ]among individuals, then meetings of infected and susceptible individuals will be proportional to the product S*I. Therefore, the rate of change of of all 3 populations (S, I and R) is shown in Fig 2, where β is the probability of transmission per contact between infected and susceptible persons, c is the contact rate and D is the duration of infectivity. The pool of infected individuals will grow as new individuals become infected (at rate +βcSI) and decline as infected individuals recover (I/D). Recovery removes individuals from the infected pool at rate I/D. Because S+I+R=1.0, we only really have to keep track of two classes (and then we know what the third must be) (Stratton, 2010). For example, if dI/dt is high then the number of people becoming infected is rapidly increasing. When dI/dt is zero then there is no change in the numbers of people becoming infected (number of infections remain steady). When dI/dt is negative then the numbers of people becoming infected is decreasing. The constants β, c and D are chosen depending disease being modelled.
The Epidemiological Parameter R0[image: ]: One fundamental parameter governs the spread of diseases, and is also related to the long term behavior and the level of vaccination necessary for eradication. This parameter is called the basic reproductive ratio R0 which is defined by epidemiologists as ‘the average number of secondary cases caused by an infectious individual in a totally susceptible population’ (Kucharski, 2014). As such R0 tells us about the initial rate of increase of the disease over a generation. When R0 is greater than 1, the disease can enter a totally susceptible population and the number of cases will increase, whereas when less than 1, the disease is will always fail to spread. Therefore, in its simplest form R0 tells us whether a population is at risk from a given disease. Some of the R0 values for different diseases are shown above. This shows how an airborne disease like measles is very infectious â and how malaria is exceptionally hard to eradicate because infected people act almost like a storage bank for the parasites inside the vector mosquitoes.
Determinants of R0 and application to epidemic modelling: For a pathogen with direct person-to-person transmission
R0 = βcD (where β is the probability of transmission per contact between infected and susceptible persons, c is the contact rate and D is the duration of infectivity).
The big questions for an epidemic are under what conditions will disease spread? (When is dI/dt>0)? When will the infection stop growing (dI/dt=0) (the epidemic is over)? What is the total fraction of the population that will contract the disease? Under what conditions will the disease spread? dI/dt is zero when I is zero (the disease is not present) or when βcS=1/D. Rearranging S =1/βcD (Stratton, 2010)
That means that there is a critical proportion of susceptible individuals that is required in order for there to be an epidemic. If S is less than that number, dI/dt< 0 and the infection will die out. Is it possible to reduce the number of susceptible individuals in the population and how? [bookmark: ref1191735]HERD IMMUNITY : (Willingham and Helft,2014) One way to prevent the spread of disease is to vaccinate individuals. If a high enough proportion of the population is vaccinated, then the epidemic will eventually die out, even in unvaccinated individuals. That is known as âherd immunityâ. Unvaccinated people can indirectly benefit if enough others in the population receive the vaccination. Herd immunity, also called community immunity, is a state in which a large proportion of a population is able to repel an infectious disease, thereby limiting the extent to which the disease can spread from person to person. Herd immunity can be conferred through natural immunity, previous exposure to the disease, or vaccination. An entire population does not need to be immune to attain herd immunity. Rather, herd immunity can occur when the population density of persons who are susceptible to infection is sufficiently low so as to minimize the likelihood of an infected individual coming in contact with a susceptible individual. Herd immunity can prevent sustained disease spread in populations, thereby protecting susceptible individuals from infection. It is applicable, however, only to infectious diseases that can be spread by human contact. The percentage of the population that must be immune to produce herd immunity differs for each infectious disease. A disease that is highly contagious, such as measles, requires a higher proportion of immune persons to achieve herd immunity than a disease that is less contagious, such as tuberculosis. In addition, individual- and population-level characteristics that influence disease spreadâsuch as susceptibility, demographics, and social habitsâaffect herd immunity. [bookmark: ref1191736][image: ]MATHEMATICAL RELATIONSHIP BETWEEN R0 AND HERD IMMUNITY: (Scherer and McLean, 2002) Herd immunity is an important consideration for mass vaccination practices. Even if a cheap, safe, and effective vaccine exists, resource, logistical, and societal constraints can prevent the vaccination of 100 percent of the population (Willingham and Helft, 2014). A reasonable target level of vaccination may be to achieve the threshold level of herd immunity (H), which is calculated as H > 1 â 1/R0, where R0 is the basic reproductive rate (Keeling, 2001). Mass vaccination can be successful through principles of herd immunity. Although disease outbreaks can still occur, they generally do so to a lesser extent than if herd immunity had not been achieved.
MODELING MEASLES: (IB Math Resources, May 2014). With measles we have an average infection of about a week, (so if we want to work in days, D=7) and R0 = 15 then
R0 = βcD 15 = βc7 βc = 2.14
Therefore our 3 equations for rates of change become:
dS/dt = -2.14 I S
dI/dt = 2.14 I S â 0.14 I
dR/dt = 0.14 I
[image: ][image: ]Unfortunately these equations are very difficult to solve â but luckily we can use a computer program to plot what happens.  We need to assign starting values for S, I and R â the numbers of people susceptible, infectious, recovered (immune) from measles. Letâs say we have a total population of 11 people â 10 who are susceptible, 1 who is infected and 0 who are immune. This gives the outcome shown in Fig 3. This shows that the infection spreads incredibly rapidly â by day 2, 8 people are infected. By day 10 most people are immune but the illness is still in the population, and by day 30 the entire population is immune and the infection has died out.
[image: ]An illustration of just how rapidly measles can spread is provided by Figure 4. This time we start with a population of 1000 people and only 1 infected individual â but even now, within 5 days over 75% of the population are infected.
Figure 5 shows the power of herd immunity. This time there are 100 susceptible people, but 900 people are recovered (immune), and there is again one infectious person. This time the infection never takes off in the community â those who are already immune act as a buffer against infection.
CONCLUSIONS: In the battle against disease, the difference between a raging epidemic and a passing fever comes down to a single number-R0. The reproduction number is useful because it captures all the processes that influence transmission, from social behavior to severity of infection, into a single measurement. The size of this number can reveal what will happen during an outbreak (Kucharski, 2014). The ability to make predictions about epidemics is one of the major strengths of mathematical approaches. It is useful to model and understand the dynamics of disease for multiple reasons: it is important for basic science as well as for public policy. Modeling of infectious diseases is helpful for prevention and control of emerging diseases like SARS and Ebola. Using models, we can test control strategies without interfering with the real world. We can therefore compare treatments and interventions before putting them into practice. By viewing epidemics as a dynamic process, we can also evaluate potential control measures. For example, we can ask are there any scenarios in which vaccines should be targeted to certain groups or whether there are scenarios in which the predicted epidemic will exceed medical capacity. It is in this role that models, of various levels of complexity, could have the greatest public-health benefit.