Negotiation and Batna
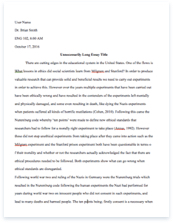
- Pages: 13
- Word count: 3136
- Category: Management Negotiation
A limited time offer! Get a custom sample essay written according to your requirements urgent 3h delivery guaranteed
Order Now1. Introduction
The purpose of this paper is to tie together various existing material on negotiation, and propose a quantitative framework, based on existing research concepts, for carrying out negotiations. In addition to developing a framework and the associated mathematical theory, we perform simulations to explore the efficacy of negotiating for more than one alternative at the same time. Negotiation is a way for parties to reach agreement in a dispute or in making a joint decision. In general, negotiations involve one or more issues that need to be settled between two or more involved parties (Raiffa, 1982). Table 1 shows a typology of the kinds of negotiations possible based on the number of negotiable issues and the number of parties involved. In the paper we consider two-party, one-issue negotiations. Table 1.
A classification of negotiation problems
|Parties |Negotiable issues | |[pic] |[pic] | | |One negotiable issue |Many negotiable issues | | |[pic] |[pic] | |Two parties |Two parties, one issue |Two parties, many issues | |Many parties |Many parties, one issue |Many parties, many issues |
Full-size table
View Within Article
The Best Alternative To a Negotiated Agreement (BATNA) introduced by Fisher et al. (1991) is the best a negotiator can do if negotiations fail, or if the negotiator walks away from a negotiation. A negotiator can and should terminate a negotiation if his BATNA is better than the likely outcome of the negotiation. A negotiatorās BATNA may change as negotiations go on. Suppose you want to buy a house. You prefer house A, and your second choice is house B. Suppose further that you decide to negotiate on house C (which is currently less attractive than house A and house B); then house A is your BATNA. If you negotiate and make the terms of house C more attractive than that of house A, then house C becomes your most preferred and your BATNA. But suppose that in negotiating for house C, you cannot get the terms more attractive than that of house A. Then you should walk away from the negotiations for C. During the process if house A is sold, house B becomes your BATNA which is more attractive to you than house C.
The BATNA serves as a reference point ([Kahneman and Tversky, 1979], [Tversky and Kahneman, 1991] and [Tversky and Kahneman, 1992]) for potential negotiations on other houses. Raiffa et al. (2002, p. 221 and p. 447) described something similar for multi-issue negotiations, which they called intertwining negotiations. By looking at the BATNA as a dynamic measure of negotiating strength, as a mechanism for deciding whether and/or when to negotiate, we develop an approach to negotiations. For simplicity, we consider only the buyer in a house-buying problem. The process of buying a house may be viewed as a one-to-many negotiation problem, in which there is one buyer and many sellers.
We may also consider the negotiation process to be made up of many two-party (buyer and seller) negotiations. A similar analysis may be made from the perspective of the seller. However, the seller is normally less able to find buyers than the buyer is able to find sellers. We might say that the house-buying problem is similar to the secretary (or spouse selection) problem. The secretary problem assumes that one considers a sequence of possible secretaries, and either chooses or rejects each candidate in succession. Once a candidate is rejected, he/she is no longerĀ available. However, the house-buying problem (or a real secretary problem for that matter) is different, because it may be possible to go back to a previously rejected alternative. We develop a prescriptive strategy for a party (a buyer or a seller, for example) in a negotiation process. Our idea is to filter alternatives systematically to help a negotiator focus on potentially āgoodā alternatives and further help the negotiator set up and manipulate a reservation price for different alternatives by considering the partyās BATNA as a reference point. The remainder of this paper is organized as follows.
After a literature review in Section 2, we present our general negotiation framework in Section 3. We consider using the Aspiration-level Interactive Model (AIM) ([Lotfi et al., 1992], [Wang and Zionts, 2005] and [Wang and Zionts, 2006]) and a Multiple Additive Value (MAV) function (Keeney and Raiffa, 1976) to help the negotiator explore the alternatives in Section 4. We also discuss the case in which the negotiator starts the negotiation process with a preliminary choice. In Section 5 we present and discuss our simulation results. In Section 6, we summarize our research and consider possible further research. 2. Background
The concept of reference point was introduced in prospect theory ([Kahneman and Tversky, 1979] and [Tversky and Kahneman, 1992]). Empirical studies demonstrate that the reference point and the framing used affect the outcome of negotiations ([Bazerman et al., 1985] and [Nagel and Mills, 1989]). See also (Carnevale and Pruitt, 1992), (Van Poucke and Buelens, 2002), (Yukl, 1974), (Kristensen and Garling, 1997) and (Raiffa et al., 2002), in which the researchers showed how a reservation price plays an important role in negotiations. In most negotiations, a party not only evaluates an offer, but also makes a counteroffer, in which an anchoring-and-adjustment process may be involved (Kahneman, 1992). Adjustments are made to generate counteroffers from an initial offer, an anchor point. A counteroffer differs depending on the anchor point chosen (Kristensen and Garling, 2000).
Northcraft and Neale (1987) showed that estimates of the value of a house made by real-estate agents and students differ depending on information about the list prices given. While an anchor point affects the counteroffers negotiators make, a reference point determines how an offer is perceived ([Northcraft and Neale, 1987] and [Kahneman, 1992]). Decision making is always an important part inĀ negotiation. In general, there are five steps in a typical decision making process. They include identifying the problem, clarifying objectives, generating alternatives, evaluating consequences, and making tradeoffs in the process of making a decision. Keeney (1992) proposed value-focused thinking, a comprehensive framework based on a methodology that helps people to think intelligently about their value system and problem. The objective of value-focused thinking is to clarify the distinction between alternatives and objectives, structure objectives qualitatively and quantify them.
The PrOACT way of thought is a systematic divide-and-conquer approach introduced by (Hammond et al., 1999) and (Raiffa et al., 2002). Moore (2005) presented a scoring system, which essentially employs an MAV function, to help a negotiator select the best alternative during a negotiation. The bounded rationality and satisficing decision model introduced by Simon (1976) involves setting aspiration levels for objectives that the decision maker would like to achieve, and then obtaining feedback about their feasibility. Incorporating these concepts taking into consideration human behavior in negotiation, we develop a negotiation strategy for a negotiator. This strategy tries to avoid some behavioral traps such as the anchoring trap and the status quo trap that exist in a negotiation process (Raiffa et al., 2002, p. 35). 3. General framework
Let us assume that the negotiator is a person who wants to buy a house. There is a set of houses available. For convenience, we consider price to be the only negotiable issue. By exploring alternatives and negotiating price with prospective sellers, the buyer makes a final decision and hopefully consummates a sale. A systematic negotiation procedure is proposed as follows (Fig. 1): 1. Problem structure: Develop a set of alternatives and criteria and an associated decision matrix, in which rows represent alternatives and columns represent criteria. The issue to be negotiated (here price is the only negotiable issue) is one of the criteria. Without loss of generality we assume that all criteria other than price are to be maximized, and price is to be minimized. Some criteria may be qualitative, although this may cause problems with the evaluative metrics, though not with one of our proposed methods.
The size of the matrix is m Ć n, where m is the number of alternatives and n is the number of criteria. We denoteĀ Ai=(ai1,ai2,ā¦,ain) as the ith alternative, where aij is the performance of alternative i on criterion j, j = 1, ā¦, n. For convenience, price is the last criterion, and we also denote the price of alternative i as pi. The alternatives are from different sellers. 2. BATNA determination: Choose the most preferred solution (the BATNA) from a set of alternatives. We consider two ways in which this may be done: a. The negotiator uses a value function, which may be either AIM that uses a nonlinear value function or an MAV, to rank alternatives and consequently determine the BATNA. b. The negotiator may somehow simply choose a most preferred alternative. 3. Filtering of alternatives: Determine the price reduction of an alternative necessary to make it more attractive than the BATNA.
If an alternative can not be made more attractive than the BATNA by price reduction, or if the minimum price reduction necessary to make it more attractive than the BATNA is believed to be excessive, then such an alternative may be eliminated from further consideration. The necessary price reduction may be determined either by assuming a value function, or subjectively by the negotiator. 4. Negotiation: Negotiate with the appropriate parties on some alternatives that survive the filtering process to determine how much they are willing to lower their price. 5. Adjustment and anchoring: Rank the alternatives using updated prices, and choose the most preferred as the new BATNA. 6. Iteration: Repeat steps 3ā5 until an agreement is reached. The process may also be terminated when improvement at successive iterations becomes sufficiently small.
Full-size image (21K)
Fig. 1. Flowchart of a negotiation framework.
View Within Article
We discuss the details of steps 2 and 3 in Section 4 and Section 5. 4. BATNA determination and filtering of alternatives
In this section, we discuss the steps of the BATNA determination and the filtering of alternatives with two methods, AIM using a Tchebycheff metricĀ and MAV. Other metrics may be used if desired. The BATNA serves as a reference point that may be used to determine a reservation price for other alternatives. 4.1. Definitions
We now introduce several definitions. For convenience, we assume all criteria are to be maximized (price replaced by its negative). Dominance: Alternative i dominates alternative k if aij[pic]akj for j = 1, 2, ā¦, n with a strict inequality for at least one j. C
Remark
The concept of convex dominance is that an alternative is dominated by a convex combination (or blend) of other alternatives. 4.2. Changing dominated to nondominated solutions by reducing price Provided that all criteria are included in a decision problem, we can always find a better alternative than a dominated alternative. There are two reasons to consider dominated solutions: (1) we may be able to change one or more aspects of a dominated alternative to make it nondominated; and (2) we may have other criteria that need to be included. In the latter case, such criteria should be defined and used in the analysis. Consider the example of Table 2 plotted in Fig. 2. There are 8 alternatives, evaluated on two criteria, quality on the vertical axis and price (inverted scale) on the horizontal axis in Fig. 2.
The buyer wants to minimize price (which is negotiable) and maximize quality. As we can see from Fig. 2, alternatives 1, 2, 3, 5, and 7 are nondominated. Alternative 3 dominates 4, alternative 5 dominates 6, and alternative 8 is dominated by the alternatives other than 7. To make alternative 4 nondominated, we must reduce its price to less than p3, i.e., just below the price of alternative 3. Similarly, to make alternative 6 nondominated, we must reduce its price to less than p5. We may also reduce the price of alternative 8 to make it nondominated.
Proposition 1
To make an alternative k nondominated, the price of k should be decreased to just less than the minimum price of all dominating alternatives, i.e., p=mini[pic]Spi-ε, where S is the set of alternatives that dominate k, and ε is a sufficiently small positive number. Proof
The results follow directly from the definition of dominance.
4.3. Convex dominance and a continuous Pareto-optimal frontier Suppose thatĀ we permit blending of solutions (the reason for doing this will become clear below). Then the Pareto-optimal frontier would be continuous. Our set of solutions is the set of convex combinations of all alternatives. Consider again the example of Table 2. The set of solutions is the convex set defined by the extreme points 1, 3, 5, 7, and 8 in Fig. 3, and the Pareto-optimal frontier consists of the line segments 1ā3, 3ā5, and 5ā7. Alternative 2, though not dominated, is convex dominated. It is dominated by a convex combination of alternatives 1 and 3. How much should we reduce the price so that dominated and convex-dominated alternatives are moved to the Pareto-optimal frontier? We may reduce the price of alternative 2 to [pic]or less to make it Pareto-optimal. Similarly, we may reduce the price of alternatives 4, 6, and 8 to make them Pareto-optimal so that they may maximize an additive value function. See Fig. 3.
Full-size image (15K)
Fig. 3. Making dominated and convex-dominated solutions nonconvex dominated.
View Within Article
In order to determine the price reduction necessary to make a dominated or convex-dominated solution nonconvex-dominated, we solve a linear programming problem:
where alternative k is dominated or convex-dominated.
Our ideas share some elements of data envelopment analysis (DEA) (Charnes et al., 1978). DEA uses a similar analysis to come up with a measure of efficiency of an alternative with various input and output measures. Our concern is to choose the best alternative subject to price negotiation.
Proposition 2
The price change for a solution to be non-convex-dominated is at least as much as the price change necessary to achieve nondominance. ProofĀ Dominance may be regarded as a special case of convex dominance. Supposing that alternative i dominates alternative k, we can write this in a convex-dominance form as [pic]. Thus any dominated alternative is convex-dominated. However, convex-dominated alternatives are not necessary simply dominated. Alternative 2 in Table 2 is one such example. ā” 4.4. Using AIM
In this section, we propose using AIM to help a user choose a most-preferred alternative. AIM uses Simonās concept of satisficing ([March, 1994] and [Simon, 1976]), as well as other concepts of Multiple Criteria Decision Making (MCDM). One of the advantages of AIM is that a convex-dominated alternative may be the most preferred ([Lotfi et al., 1992] and [Wang and Zionts, 2006]). The ranking of alternatives in AIM is determined using a scalarizing function defined by Wierzbicki (1980).
The highest-ranked alternative for an aspiration level is a sufficiently-defined nearest feasible nondominated alternative. It may be thought of as a variation of goal programming (Charnes and Cooper, 1961). Although there are a number of different types of scalarizing functions possible (Wierzbicki, 1980), we choose a Tchebycheff metric as our scalarizing function. We consider two types of criteria: price to be minimized, and others to be maximized. (The AIM method can handle more than two types of objectives, but we will not consider them further here. Nonetheless, the analyses hold for other types of criteria as well.) Let αj denote a negotiatorās aspiration level on criterion j. We defineĀ which is the ideal point of criterion j, j = 1, ā¦, n.
which is the nadir point of criterion j, j = 1, ā¦, n. The feasible range of the aspiration level for criterion j is considered to be between Ij and Nj.Ā We assume the ideal point has a sufficiently low price so that it is invariant to any alternativeās price adjustment. We use a normalization process to determine the weights based on the aspiration level.
Given an aspiration level, the weights are fixed. The score γi for alternative i is determined to beĀ where Ļ is a sufficiently small positive scalar, and [pic]. Note that [pic]is a tie-breaking term and only applied when the two alternatives i, k have minj=1,ā¦,nwjĪ“ij=minj=1,ā¦,nwjĪ“kj. The alternative that has the highest score is the most preferred. In this section we assume that the negotiatorās aspiration level is given. Consider the example (Table 2) shown in Fig. 4a and b in which two different aspiration levels are given. The aspiration levels are located at the intersection of the dashed lines. For both aspiration levels, alternative 3 should be the most preferred in AIM and therefore be the negotiatorās BATNA. In the case of Fig. 4a, three alternatives (1, 2, and 4) can possibly be made better than alternative 3.
If alternative 1 or 2 can have its price reduced to just less than that of 3, such an alternative would dominate 3. This is a sufficient condition to guarantee that alternative 1 or 2 can be made superior to the BATNA. However, a smaller price reduction may be all that is needed to make alternative 1 or 2 (but not 4) preferable to the BATNA. The level of price reduction necessary depends on the aspiration level the negotiator has. Given the aspiration level in Fig. 4b, only alternatives 1 and 2 may be made superior to alternative 3, but alternative 4 can not be made superior to alternative 3. Further alternatives 1 and 2 need less price reduction than that needed in Fig. 4a. A similar analysis follows for the case of three or more criteria.
Proposition 3
Let Cn=In-Nn, wn be the weight for price as defined in Eq. (5), and γk be the score for the BATNA k as defined in Eq. (6). (1) An alternative i other than the BATNA that has [pic]has a price pi not less than [pic]. Or, (2) An alternative i other than the BATNA that has a price pi lower than [pic]has [pic]. Proof
(1) Assuming [pic]. Following the condition and recalling Cn< 0, we have [pic]. Thus [pic]follows [pic], which is a contradiction to k being the current most preferred. (2) This is a corollary of (1). ā”
Proposition 4
Let Ī·i=minj=1,ā¦,n-1wjĪ“ij, where wj, and Ī“ij are defined in Eq. (6).
(1) An alternative i that has [pic]can be made superior to the BATNA k by reducing its price to less than [pic]. (2) Given Ļā 0, in order to make the alternative i that has [pic]superior to the BATNA k, we need to reduce its price to less than [pic], where [pic]is as in (1), and [pic]. (3) Given Ļ = 0, only alternatives that have Ī·i>γk can be made superior to the BATNA k by reducing price to less than [pic]. An alternative that has Ī·i