Siwes Industrial Training Report
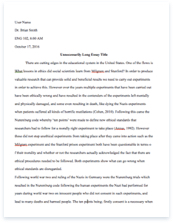
- Pages: 27
- Word count: 6619
- Category: College Example Management
A limited time offer! Get a custom sample essay written according to your requirements urgent 3h delivery guaranteed
Order Now1.0INTRODUCTION
The students industrial work experience (SIWES) is a skill training program designed to expose and prepare students of higher institution for the working environment they are likely to meet after graduation. SIWES was established by industrial training fund (ITF) in 1973 to solve the problem of lack of adequate practical skills, in preparation for employment in industries by Nigeria graduates. The SIWES program runs in the Nigeria universities in conjunction with the industrial training fund unit, to promote practical in tertiary institution. The aim of the program is to bridge the gap existing between theoretical aspect of what is being taught in the lecture rooms and what is actually obtained in the field it is aimed at exposing students to challenges they are likely to come across upon their graduation from the universities and to adequately expose students to professional work methods. Participation in Industrial training is a well-known strategy. Classroom studies are integrated with learning through hands-on work experiences in a field related to the studentâs academic major and career goal.
It enhances an experiential learning process that not only promotes career preparation but also provides opportunities for learning, to develop skills necessary to become leaders in their chosen professions. Participation in SIWES has become a necessary pre-condition for the award of Diploma and Degree certification in specific discipline in most institutions of higher learning in the country, in accordance with the educational policy of government. OPERATORS OF THE SIWES PROGRAM: The industrial training fund (ITF), employers of labour, the higher institutions and some coordinating agencies like Nigeria university commission (NUC), (NCCE) and (NBTE) are the operators of this program. FUNDING: The Federal Government of Nigeria fund this program BENEFICIARIES: Undergraduate students of the following: Agriculture, Engineering Technology, Environmental, Science, Medical Science and pure and Applied Sciences DURATION: Four month for Polytechnics and Colleges of Education, and Six months for the Universities 1.1 OBJECTIVES OF SIWES
1.It provides an avenue for students to acquire industrial skills and experiences in their course of study. 2.It prepares students for the work situation they are likely meet after graduation. 3.It develops the skills and techniques directly applicable to their careers. 4.It makes the transit from universities to the labour market easier, and thus enhances students contact for later job placement. 5.It provides student with an opportunity to apply their theoretical knowledge in real situation, thereby bridging the gap between world and the practical day to day environment. 6.It enlists and strengthens employersâ involvement in the entire educational process of preparing university graduates for the employment in the industry. 7.SIWES increases a studentâs sense of responsibility.
8.It affords student the opportunity to develop attitudes conducive to effective interpersonal relationship 9.SIWES affords students the opportunity to acquire good work habits. 10. SIWES helps students integrate leadership development into experiential learning process.
1.2 HISTORY OF THE INSTITUTE (I, A, R & T) The institution of agriculture research and training (IAR & T) Obafemi Awolowo University is a multi commodity institute for research, services and training for agricultural development in Nigeria The pioneer school of agriculture in Nigeria was established on moor plantation in 1921.The establishment of the college of animal health and production technology, Ibadan followed in 1964.The three colleges and research divisions of the western region ministry of agriculture and natural resources became the institute of agriculture research and training, Ibadan in 1969.Following a charter signed by the vice chancellor of the university of Ife (now Obafemi Awolowo University) and the governor of the former western region of Nigeria. IAR & T serves the needs of the Nigerian farmers in general and farmers in southwestern Nigeria in particular within the context of its integrated agricultural resources, development and training strategy.
The institute has 560 staff members 1.3 MISSION AND FOCUS OF THE INSTITUTE To serve as a national center for integrated improvement of the genetic potential yield, utilization and nutritional qualities of major foods and agro-industrial crops as well as livestock breeds adapted to the broad agro-ecological zones of southwestern Nigeria. IAR & T investigates, evaluate, develops and promotes farming systems based on improved technologies aimed at increasing and maximizing the overall agricultural productivity and production.IAR & T provides recent junior, intermediate and high level manpower training for national agriculture development. The institute also collaborates with other universities, national, regional and international institutions in the practical application and adoption of improved agricultural production technologies.
The institute has six research programs which are land and water resource management program, cereal improvement program, farming system research and extension program, industrial crop improvement program, livestock improvement program and grain legumes improvement program. It also comprise of four service units namely; crop production unit, library and documentary unit, information technology unit and bora agro ventures unit.IAR & T is located in Ibadan the capital city of Oyo state and has seven sub stations (Ikenne, Ilora,Akure, Ballah,Orin Ekiti,Ile ife and Kisi) with experimental sites in Amakama, Umuahia in Abia state, Niger state, Eruwa in Oyo state as well as adopted villages for technology dissemination in Moloko-Asipalogun in Ogun state and Oniyo-Ogbomoso in Oyo state
1.4 ORGANISATION CHART
1.5 INFORMATION & TECHNOLOGY UNIT
The Information and Technology Unit was formally known as Statistics and Computation Unit until 2004. The Information Technology Unit (ITU) is the gateway of the Institute to the global village and the success of the Institutesâ technology generation and dissemination efforts depends to a large extent on the active support of the unit. 1.6 ORGANIZATION OF INFORMATION & TECHNOLOGY UNIT
The activities of ITU revolve around five sections. These are: . Biometrics and agro meteorology.
. Experimentation and statistical Analysis. . Computer Services.
. Applied Research and Training.
. Administrative Services.
1.7 Experimentation Statistical Analysis Experimentation and statistical Analysis is a section under Information Technology Unit, with activities ranging from: ďś Experimental Design.
ďś Analysis of Statistical Data (primary data).
ďśInterpretation of Analyzed Results.
ďśProcess of questionnaire for Analysis.
ďśDocumentation of Analyzed Statistical Data (secondary data).
CHAPTER TWO
2.0Introduction:
Decision makers make better decisions when they use all available information in an effective and meaningful way. The primary role of statistics is to provide decision makers with methods for obtaining and analyzing data, make inferences that will help in decision making. Statistics is used to answer long-range planning questions, such as when and where to locate facilities to handle future sales. Statistics deals with both numerical and non-numerical data, the aim is to provide meaningful information from the data being analyzed and to provide possible guess about future, and this can be done with the help of some statistical tools or formulae which depends on the assumptions followed by the data. Due to the difficulty in carrying out census, statisticians depend majorly on sample which is the subset of the population and use the information obtained about the sample (RANDOM SAMPLING) to infer conclusion about the whole. Statistics as a course consist of different aspects ranging from collection, organizing, summarizing, presenting, analyzing, and interpreting of data to get meaningful information.
Each of the aspect is very vital before meaningful information about any research can be reached. Statistics are used in the scientific study of agriculture as a tool to determine if the differences in variables are real or due to chance. This translates to the farmer to let him know with confidence which varieties are better than other varieties or which fertilizer treatments will give better yields than others. The use of statistical analysis and experimental design was quickly embraced in all areas of agricultural science because of the ability to interpret the results correctly and then to successfully (and confidently) apply this newly garnered information to solving real world problems. Many scientists will acknowledge that without this connection the adaptation of new agricultural knowledge would have been much slower and there is no way to understand how dramatic that effect would have been seen in the world at large.
2.1 SOME DEFINITIONS
Statistics has been defined as a scientific ways of dealings with numbers, is a science that deals with collection, organizing, summarizing, presenting, analyzing and interpretation of data in such a way to minimize any uncertainty and to be able to put degree to such uncertainty. Statistics are measurement, enumerations or estimates of natural or social phenomena systematically arrangement to exhibit their inner relation. By Statistics we mean quantitative data affected to a marked extend by a multiplicity of causes. The science of Statistics is essentially a branch of applied mathematics and can be regarded as a mathematics applied to observation. Statistics as a course is divide into two types, these are 2.11 Descriptive Statistics:
Descriptive statistics allow a scientist to quickly sum up major attributes of a dataset using measures such as the mean, median, and standard deviation. These measures provide a general sense of the group being studied, allowing scientists to place the study within a larger context. Descriptive Statistics is the type of statistics that deals with part of population without making inference about the whole population. 2.12 Inferential Statistics:
Inferential statistics are used to model patterns in data, make judgments about data, identify relationships between variables in datasets, and make inferences about larger populations based on smaller samples of data. It is important to keep in mind that from a statistical perspective the word âpopulationâ does not have to mean a group of people, as it does in common language. A statistical population is the larger group that a data set is used to make inferences about â this can be a group of people, corn plants, meteor impacts, oil field locations, or any other group of measurements as the case may be. 2.13 VARIABLES
Variables are quantities or qualities that may assume any one of a set of values.
Variables may be classified as nominal, ordinal, interval and ratio
1. Nominal variables use names, categories, or labels for qualitative values. Typical nominal variables include gender, ethnicity, job title, And so forth.
2.Ordinal variables: Like nominal variables are categorical variables. However, the order or rank of the categories is meaningful. For example, staff members may be asked to indicate their satisfaction with a training course on an ordinal scale ranging from âpoorâ to âexcellent.â Such categories could be converted to a numerical scale for further analysis.
3. Interval variables are purely numeric variables. The nominal and ordinal variables noted above are discrete since they do not permit making statements about degree, e.g., âPerson A is three times more male than person Bâ or âPerson A rated the course as five times more excellent than person B.â Interval variables are continuous, and the difference between values is both meaningful and allows statements about extent or degree. Income and age are interval variables.
4.Ratio scale: have the properties of interval scale and true zero point. 2.2 Statistical Data:
Data (the plural form of the word datum) are scientific observations and measurements that once analyzed and interpreted can be developed into evidence to address a question. Data lie at the heart of all scientific investigations, and all scientists collect data in one form or another. Ungrouped Data: Data which have been arranged in a systematic order are called raw data or ungrouped data. Grouped Data: Data presented in the form of frequency distribution is called grouped data. 2.21
Sources of Data
There are two types (sources) for the collection of data. (1) Primary Data
(2) Secondary Data
ďPrimary Data
The primary data are the first hand information collected, compiled and published by organization for some purpose. They are most original data in character and have not undergone any sort of statistical treatment. Example, Population census reports are primary data because these are collected, complied and published by the population census organization. ďSecondary Data
The secondary data are the second hand information which is already collected by someone (organization) for some purpose and are available for the present study. The secondary data are not pure in character and have undergone some treatment at least once. Example: Economics survey of Nigeria is secondary data because these are collected by more than one organization like Bureau of statistics, Board of Revenue, the Banks etcâŚ
2.22 Collection of data
statistical data can be obtained through:
ďPersonal Investigation: The researcher conducts the survey him/herself and collects data from it. The data collected in this way is usually accurate and reliable. This method of collecting data is only applicable in case of small research projects.
ďThrough Investigation: Trained investigators are employed to collect the data. These investigators contact the individuals and fill in questionnaire after asking the required information. Most of the organizing implied this method. ďQuestionnaire: The researchers get the data from local representation or agents that are based upon their own experience. This method is quick but gives only rough estimate.
2.3 DESIGN OF QUESTIONAIRE
Questionnaire is a list that contains lists of questions that respondents are to answer and it should be design in such a way to encourage respondent. The various form of Questionnaire includes:
1.The Dichotomous
2.The Open Ended Type
3.The Multiple Choice
2.31 The Dichotomous
This is the simplest form of Questionnaires and it requires the respondents to choose between the responses e.g. â˘Do you go to school everyday
Yes ( ) No ( )
â˘Do you agree with this statement
Yes ( ) No ( )
Although the dichotomous is easy to construct, it sometimes over simplifies an issue and does not give room for compromise 2.32 The Multiple Choices
The multiple choices are more appropriate in certain situation. For example, in a public opinion survey, we could ask the following question â˘Is female students brilliant than male students
Strongly agree ( )
Agree ( )
Undecided ( )
Not agree ( )
Strongly disagree ( )
2.33 THE OPEN /END TYPE
This is a type of questionnaire that allows a person, the must freedom of responses. For example, the type of question below requires an open / end answer. What is your opinion about Nigeria continuing with the devaluation of Nigeria programmed? The obvious disadvantage of such questionnaire is the difficulty in classifying the results or the responses. No matter the type of questionnaire being used, question must be simple and phrased to give the same meaning to all persons. Principal points in Designing Questionnaires. 1.The number of questions should be kept at a minimum
2.Questions should be short and clear
3.Offensive question should be avoided
4.Influential or misleading question should not be used
5.Questions should be easy to answer
6.Questions should require simple answer
Characteristics of a good questionnaire
1.A good questionnaire should not be ambiguous
2.A good questionnaire must be easily understood
3.A good questionnaire should not require that tedious calculation be made
4.It should cover the exact object of the inquiry
5.A good questionnaire should not be too long.
ďThrough Telephone: The researchers get information through telephone; this method is quick and gives accurate information. 2.4 Sorting of Data Sorting arranges data into either alphabetical or numerical order. For example, a list of parts might be sorted by part number, and a list of experimental unit might be sorted by last name. You normally sort alphabetic information (store names, customer names, and so on) into alphabetical order; you sort numeric information (sales figures, quantity of units sold, and so forth) into numerical order. In either case, you can sort the data from Low to High which is A to Z or 1 to 10, or High to Low–Z to A or 10 to 1. Sorting statistical Data: arrange data into meaningful, useful sequences so that you can easily analyze the information and prepare reports. For example, a long experimental unit name will be sorted to short meaningful name. Pivoting rows and columns: reorganizes data to gain different perspectives on it. When pivoting data, move the data from one axis to another to arrange it for efficient analysis. That is, you can move rows to become columns, or columns to become rows.
For example, you might want to view treatment and replicate or weight and length of a data side-by-side on a worksheet for easy analysis and comparison respectively. In most cases, just by pivoting the data you can quickly and easily switch between different views of the data. Drilling into and out of levels of detail: you use drilling to either consolidate data to see it at an aggregate level, or to see finer levels of detail. You drill out to get the big picture; you drill in to see the details. Drilling helps you easily locate related information in a worksheet. For example, suppose you’re analyzing data showing measurements ranging from 3 to 4. To see a measurement at a higher level, such as 45, you can drill out of that information. Totaling Data–numeric data on worksheets is presented in rows and columns. You can sum their data to produce totals and sub-totals. 2.5 ORGANIZING OF DATA:
Having obtained some quantitative information from your research, you now wish to make some findings. This quantitative information is referred to as raw data. These raw scores, more often than not are usually large thus cumbersome to handle and so you required to organize them. Organization of data brings orderliness and better perception of the data. For organizing of data the knowledge of some basic concepts are required. These include: frequency tallying of scores, class intervals, class limit, class boundaries, mid-point, coordinates etc. 2.6 ANALYSING OF DATA
Analysis of data is a process of inspecting, cleaning, transforming, and modeling data with the goal of highlighting useful information, suggesting conclusions, and supporting decision making. Data analysis has multiple facets and approaches, encompassing diverse techniques under variety of names, in different business, science, and social science domains. The analysis given to any data depends majorly on the objectives of the survey and to a greater extent on the number of variables in the survey. For example Analysis of Variance is of greater used in Agricultural research where the effect of treatments is being measured on variables, Regression and Correlation are used, when the relationship and strength of relationship between variables is to be measured. The analysis given to a data depends on the aim and the objectives of the data. There many statistical tools that are used in the analysis of data like ANOVA, t-test, correlation, regression, multivariate analysis, cluster analysis and so on.
CHAPTER THREE
3.0 introductions
The use of statistical analysis like correlation / regression and experimental design was quickly embraced in all areas of agricultural science because of the ability to interpret the results correctly and then to successfully (and confidently) apply this newly garnered information to solve real world problems. Many scientists will acknowledge that without this connection the adaptation of new agricultural knowledge would have been much slower and there is no way to understand how dramatic that effect would have been seen in the world at large.
3.1 Experimental design
In experimentation we attempt to monitor the effects of certain inputs or material on the subject matter of interest. The inputs could be different hedgerow leguminous plants planted under identical conditions, while the effects to be monitored could be the changes in soil fertility status, the yield of agricultural crops planted between the rows, the productivity of the animal being fed with the foliage from the tree crops, or the tree crop performance. The allocation of treatments (inputs) to the experimental units (plots) may be loosely referred to as the design.
3.2 Basic Concepts in Experimental Design
The knowledge of experimental design is based on some terminologies which are experimental unit, treatment, experimental error. 3.2.1 The plot or experimental unit is the smallest unit receiving a certain treatment. The information or-data for comparison are from such single units. Examples include a single animal or group of animals receiving the same feed from the same source, a small plot having the same type of trees or agricultural crops, and so on. 3.2.2 The treatment is the material being forced on the subject (unit) and whose effect is to be monitored. The treatment can be either qualitative (e.g., species, fertilizer types) or quantitative (e.g., time periods, quantified levels of a fertilizer type). 3.2.3 The experimental error is a measure of the sum of variation between plots or units receiving same treatments. Inherent variability in the subject, uncontrolled external influences, and lack of uniformity in the application of treatments are possible causes of experimental error.
Experimental error should be controlled so that we can estimate the treatment effects properly and compare effects of various treatments effectively. 3.2.4 Replication: Experiments of the same nature, when presented under similar conditions should yield similar results. In other words, researchers would want to ensure consistency in their results. The simplest way to achieve this is through the “repetition,” i.e. “replication,” of the same treatment on several plots or experimental units. Repetition on the same plot is not recommended as observations are unlikely to be independent. Moreover, the use of several small plots instead of one large plot ensures minimization of the effect of uncontrolled variability in the field. 3.2.5 Coverage or Blocking: A block is a relatively large area or several identical units receiving all or most of the treatments.
One is encouraged to “block” if one can vouch for the homogeneity within blocks and the heterogeneity between blocks. Because of the limitation of homogenous plots and the relatively large area required for alley-farming and agro forestry trials, one could also consider a location as a “block.” The distinction between “replication” and “blocking” should be evident. Blocking is another way of improving the estimation of the error term, but only if the blocking is justified. 3.2.6 Randomization: This refers to the allocation of treatments to plots in such a way that, within a specific experimental design, units are not discriminated for or against. Each unit is supposed to have the same chance of receiving a particular treatment. Randomization is a necessity as no two units or plots are exactly the same. Statistically, the randomization procedure allows elimination of bias and ensures the computation of valid sampling errors. 3.3 categories of experimental design
We generally classify scientific experiments into two broad categories, namely, single-factor experiments and multifactor experiment. In a single-factor experiment, only one factor varies while others are kept constant. In these experiments, the treatments consist solely of different levels of the single variable factor. Our focus in this chapter is on single-factor experiments. In multi-factor experiments (also referred to its factorial experiments), two or more factors vary simultaneously. The experimental designs commonly used for both types of experiments are classified as: ⢠Complete Block Designs
Completely Randomized Design (CRD)
Randomized Complete block (RCBD)
Latin Square design (LSD)
⢠Incomplete Block Designs
Lattice
Group balanced block
3.31 Completely Randomized Design (CRD)
This is the simplest design. In CRD, each experimental unit has an equal chance of receiving a certain treatment. The completely randomized design for p treatments with r replications will have rp plots. Each of the p treatment is assigned at random to a fraction of the plots (r/rp), without any restriction 3.32 Randomized complete block design Randomized Complete Block Design (RCBD) is characterized by the presence of equally sized blocks, each containing all of the treatments. The randomized block design for t treatments with r replications has rt plots arranged into r blocks with t plots in each block. Each of the âtâ treatments is assigned at random (Randomization) to one plot in each block. The allocation of a treatment in a block is done independently of other blocks. 3.33 Latin Square Design (LS)
The Randomized Complete Block design is useful for eliminating the contribution of one source of variation only In contrast, the Latin Square Design can handle two sources of variations among experimental units In Latin Square Design, every treatment occurs only once in each row and each column. The Latin Square (LS) design thus minimizes the effect of differences in fertility status within each block. The total sources of variation are made up of row, column, treatment differences, and experimental error. 3.34 Incomplete Block Designs
One precondition for both the RCB and LS designs is that all treatments must appear in all blocks and all rows (For RCB) or columns (For LSD). Sometimes with large number of treatments (say 20 accessions), each requiring relatively large plot sizes, this condition may not be practicable. Latin Square and RCB then fail to reduce the effect of heterogeneity(s). The designs in which the block phenomenon is followed but the condition of having all the treatments in all blocks is not met are called Incomplete Block designs. In Incomplete Block situations, the use of several small blocks with fewer treatments results in gains in precision but at the expense of a loss of information on comparisons within blocks. The analysis of data for incomplete block designs is more complex than RCB and LSD. Thus where computation facilities are limited, incomplete block designs should be considered a last resort.Among incomplete block designs, lattice designs are commonly used in species and variety testing.
3.4 Correlations
Correlation is a statistical tool which studies the relationship between two variables and Correlation Analysis involves various methods and techniques used for studying and measuring the extent of the relationship between the two variables. Two variables are said to be in correlation if the change in one of the variables results in a change in the other variable. 3.41 Linear and Non-linear correlation
The correlation between two variables is said to be linear if the change of one unit in one variable result in the corresponding change in the other variable over the entire range of values. The relationship between two variables is said to be non â linear if corresponding to a unit change in one variable, the other variable does not change at a constant rate but changes at a fluctuating rate . 3.42 Positive and Negative Correlation If the values of the two variables deviate in the same direction i.e. if an increase (or decrease) in the values of one variable results, on an average, in a corresponding increase (or decrease) in the values of the other variable the correlation is said to be positive. Correlation between two variables is said to be negative or inverse if the variables deviate in opposite direction. That is, if the increase in the variables deviate in opposite direction. That is, if increase (or decrease) in the values of one variable results on an average, in corresponding decrease (or increase) in the values of other variable. . A correlation of zero means there is no relationship between the two variables.
3.43 Coefficient of Correlation
One of the most widely used statistics is the coefficient of correlation ârâ which measures the degree of association between the two values of related variables given in the data set. It takes values from + 1 to â 1. If two sets of data have r = +1, they are said to be perfectly correlated positively if r = -1 they are said to be perfectly correlated negatively; and if r = 0 they are uncorrelated. The coefficient of correlation ârâ is given by the formula r =
3.44 Rank Correlation
Data which are arranged in numerical order, usually from largest to smallest and numbered 1, 2, 3 —- are said to be in ranks or ranked data. These ranks prove useful at certain times when two or more values of one variable are the same. The coefficient of correlation for such type of data is given by Spearman rank difference correlation coefficient and is denoted by R. In order to calculate R, we arrange data in ranks computing the difference in rankâdâ for each pair.. When administer questionnaires, some of the variables are ranked data. I used rank correlation in such cases. R is given by the formula
Where is the square of the difference between the two ranks
3.5 Regression Analysis
Regression analysis, in general sense, means the estimation or prediction of the unknown value of one variable from the known value of the other variable, if the two variables are significantly correlated. It is one of the most important statistical tools which are extensively used in almost all sciences not Agricultural Science only. Regression analysis is a mathematical measure of the average relationship between two or more variables in terms of the original units of the data 3.51 Regression equation
Suppose we have a sample of size ânâ and it has two sets of measures, denoted by x and y. We can predict the values of âyâ given the values of âxâ by using the equation, called the REGRESSION EQUATION. y* = a + bx
Where the coefficients a and b are given by
The symbol y* refers to the predicted value of y from a given value of x from the regression equation. Simple correlation and linear regression analysis are analyzed in the next chapter using statistical packages.
3.6 DESCRIPTIVES
Descriptive statistics include the numbers, tables, charts, and graphs used to describe, organize, summarize, and present raw data. Descriptive statistics are Most often used to examine:
1. Central tendency (location) of data, i.e. where data tend to fall, as measured by the mean, median, and mode. 2. Dispersion (variability) of data, i.e. how spread out data is, as measured by the variance and its square root, the standard deviation. 3. Skew (symmetry) of data, i.e. how concentrated data are at the low or high end of the scale, as measured by the skew index. 4. Kurtosis (peakedness) of data, i.e. how concentrated data are around a single value, as measured by the kurtosis index
CHAPTER FOUR
EXPERIENCE GAINED/WORK DONE
4.0 Introductions
In the present world, information is a resource and like any other resource, its proper, efficient and effective use is the key to success. Information is extracted from data after its proper processing. We are gathering a lot of data every day by putting in considerable efforts/money. Next is the question of its utilization/processing. The huge amount cannot be processed timely and efficiently through manual methods.
Raw data handling, its tabulation and preliminary analysis is the most time consuming, cumbersome and boring task in research. So in the experimentation and statistical analysis section at the Institute of Agricultural Research and Training, we were taught different statistical packages like EXCEL, SPSS, EASY FIT, KYPLOT,WINSTAT, PAST, STATISTICAL, INSTAT, SAS, JMULTI, STATASSIT, etc. which can help in this regard, perfectly. Analysis of data in relation to descriptive statistics, test of Significance, correlation, regression, Anova and multivariate analysis etc. can be had efficiently with the help of these aforementioned packages. This chapter deals with how to make use some of these packages for simple analysis of data. 4.1 Microsoft Excel
A spreadsheet is an electronic document that stores various types of data. There are vertical columns and horizontal rows. A cell is where the column and row intersect. A cell can contain data and can be used in calculations of data within the spreadsheet. An Excel spreadsheet can contain workbooks and worksheets. The workbook is the holder for related worksheets.
4.11 Entering of Data in Microsoft Excel
When Microsoft Excel opens, at the top of the screen you will find the main menu items (Home, Data, View, Insert, Formulas, Review, Page layout, and). In the Institute of Agricultural Research and Training Ibadan, at the Experimentation and Statistical Analysis Section, we make use of Microsoft excel for the entering of the experimental data, coding of questionnaires before imported to any of the statistical packages for Analysis. The data is entered in the space called cell. 4.2 SAS (Statistical Analysis Software)
SAS Institute Inc. (pronounced “sas”]), headquartered in Cary, North Carolina, USA, has been a major producer of software since it was founded in 1976 by Anthony Barr, James Goodnight, John Sall and Jane Helwig. SAS was originally an acronym for Statistical Analysis System but, for many years, has been used as a trade name to refer to the company as a whole and its line of software products, which have since broadened beyond the statistical analysis sphere. SAS Institute is one of the largest privately-held corporations in North Carolina and in the software business. 4.2.1 Statistical Package (SAS)
At the Institute of Agricultural Research And Training Ibadan (I, A, R&T), the most preferably statistical package for researchers and the Institute (I, A, R&T) official Analysis is SAS. All other aforementioned statistical packages are used for individual researchers Analysis. It contains many built-in procedures for doing descriptive, analytic and exploratory analyses. It allows users to conduct wide range of statistical analyses, including analysis of variance, regression, categorical data analysis, multivariate analysis, survival analysis, cluster analysis, and nonparametric analysis, etc. 4.2.2 FEATURES OF SAS
SAS has two main ways of analyzing data. One way is to enter commands through the SAS command editor the other way is to use the ‘point and click’ or interactive options provided in SAS Analyst. The point and click is the easiest, and that was what we made use at the Institute (I. A. R&T). SAS has five windows. The EDITOR window is where you will write program code with SAS commands. The LOG windows tell you if your SAS commands have worked. The OUTPUT window is where the results will appear. It is very important to always check the LOG window for important notes or warnings before assuming the output is correct. The RESULT window is where you can navigate and manage SAS output. It contains a tree diagram of your output and enables you to view and print individual items. The EXPLORER window enables you to view and manage your SAS files
4.2.3 Point and click
Using this approach you do not have to remember the procedure names or the appropriate commands. While useful for most straightforward analyses, however, the point and click approach is limited if you need to perform complex data manipulation steps, or to write or invoke certain macros. Still, for most analyses, this is probably the way beginners should start. Data may be input manually in the body of the program, or they may be read in from a file (Excel). 4.2.4Â Importing Data into SAS Analyst The data to be imported into SAS analyst must have been fully entered and saved into Microsoft Excel, then open SAS for window on the computer, SAS environment is displaced, as shown below.
Go to File Import DataChoose Data format (default is Excel)Next Browse for the fileNext Create a name for your new file under member (Make sure to keep work folder unchanged)Nextyou may skip this step and click on Finish. The SAS environment will displace Data imported successfully as shown below. On the left hand side of the SAS window there is vertical sub- window called Explorer and the default shows two directories. Libraries and File. Shortcut as shown above. Double click on the Library, then work folder and locate your data file. Double click on it to view your loaded data. It should open in a new window and have the following name â VIEETABLE: WORK name of your file. In the new sub- window which must have loaded your data, go to solution then Analyst as shown below.
ďNow the data have been successfully imported in a new sub-window, ready for Analysis as shown below.
4.2.5 Experimental Data Analysis using SAS
Once the data have been loaded, they will appear in the spreadsheet. You can examine the data by using the scroll bars to move up and down and from side to side. Clicking the maximize button on the top right hand corner of the window will also allow you to see more of the data. Note that missing data are indicated by a dot. 4.3 SPSS Statistics
SPSS Statistics is a comprehensive system for analyzing data. SPSS Statistics Can take data from almost any type of file and use them to generate tabulated reports, charts, and plots of distributions and trends, descriptive statistics, and complex statistical analyses. SPSS Statistics makes statistical analysis more accessible for the beginner and more convenient for the experienced user. Simple menus and dialog box selections make it possible to perform complex analyses without typing a single line of command syntax. The Data Editor offers a simple and efficient spreadsheet-like facility for entering data and browsing the working data file.
4.4 EASYFIT
EasyFit is a data analysis and simulation application allowing fitting probability distributions to sample data, select the best model, and apply the analysis results to make better decisions. Easy Fit can be used as a stand-alone Windows application or with Microsoft Excel and other third party Excel-based simulation tools. 4.4.1 Key Features
EasyFit combines the classical statistical analysis methods and innovative data analysis techniques, making it a tool of choice for anyone dealing with probability data. Product features include: â˘support for more than 50 continuous and discrete distributions; â˘powerful automated fitting mode combined with flexible manual fitting capability; â˘interactive graphs;
â˘goodness of fit tests;
â˘random number generation;
â˘easy to use interface;
â˘Comprehensive Help system.
4.4.2 Excel Integration
Excel users can apply the power of EasyFit without the need to leave their favorite spreadsheet application. EasyFit seamlessly integrates into the main menu of Excel, allowing you to perform data analysis and simulation right in Excel. EasyFit provides more than 650 additional Excel worksheet and VBA functions which can be used to carry out advanced probability calculations such as Monte Carlo simulation.
4.5 KYPLOT
Kyplot offers you an environment for data analysis and visualization. In kyplot you can analyze data with a broad range of computing and statistical methods on a spreadsheet interface like Excel and visualization results as versatile graphs. Kyplot can serve as a presentation tool as well. Below is Kyplot main window.
KyPlot supports the following methods of data analysis.
Measurement of amplitudes and areas of peaks
Amplitudes and areas of peaks in signals can be interactively measured. 2D Fourier transform
KyPlot now supports calculation of 3D fast Fourier transform and outputs as graphs or images. Spreadsheet calculations with mathematical formulas (Excel compatible) Removal of linear trend and numerical filtering for time series data Numerical differencing, differentiation and integration
Creation of histograms, cumulative distributions, regressograms and density plots Matrix and vector operations
Numerical solutions of algebraic equations, nonlinear equations and differential equations Numerical integration of functions
Nonlinear least squares estimation and maximum likelihood estimation Linear/quadratic programming and unconstrained or constrained linear least squares estimation Numerical deconvolution
Fourier transforms
Spectral analysis
(Estimation of autoconvariance and spectral density functions by FFT, Blackman-Turkey method or AR models) Time series analysis
(ARM model, seasonal adjustment model, time-varying variance estimation, time-varying coefficient AR model, locally stationary AR model and change point estimation) Gabor (Short-time Fourier) transform
Wavelet analysis
(discrete and continuous wavelet transforms and wavelet thresholding) Simulation of random data
4.6 INTERPRETATION OF RESULT
Here we see how statistical Result is being interpreted after it has been analyzed, the interpretation given to any data depend majorly on the type of analyses done and in some cases on the HYPOTHSES being hypothesized. The data used here was not collected from any source nor was it collected from where I did my Industrial Training attachment, the aim is only to give little explanation on how some analyses is being interpreted. Here effect of five treatments are compared to see if the means are significant or not and the effect of three seasonal planting period are equally compared on the variable to see if there is any seasonal effect on the parameters or not and the interaction between the treatments and the season are equally determined using TWO WAY ANALYSIS OF VARIANCE, the correlation between the parameters are equally obtained and the descriptive. All these are done using SAS PACKAGE. For the TWO WAY ANALYSIS OF VARIANCE, the hypothesis are given by H = treatments means are not significantly different