Credit Risk Assessment in Consumer Lending
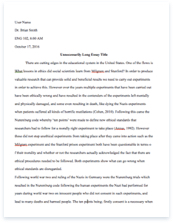
- Pages: 12
- Word count: 2862
- Category: Consumerism Risk
A limited time offer! Get a custom sample essay written according to your requirements urgent 3h delivery guaranteed
Order NowThis Point of View is focused on Credit Risk Assessment in the Consumer Lending domain including Auto, Consumer Durables and Personal loans, but excluding Mortgage. It looks at assessment using Credit Risk Modelling as well as Subjective Analysis.
INTRODUCTION
Consumer lending has been beset with severe issues since the debt crisis of 2008. Rising unemployment and decreasing repayment capabilities have brought in increased risk in credit. Consumer deleveraging has also not helped increase the lending volumes of banks.
Banks are beginning to intensify the holistic assessment of risk, risk modeling as well as creation of scoring models. Historically banks had a legacy of poor credit standards, insufficient risk management and focus on volume growth. Regulation has also played an important part in an increased focus on risk and compliance.
Consumer lending in broad business terms refers to Auto finance, Personal loans, Consumer durable loans and Mortgages. This point of view focuses on Credit Risk Assessment in Consumer lending. Credit risk in Consumer Lending is defined as the possibility that a bank borrower will fail to meet his obligations in accordance with the borrowing terms. Credit Risk Assessment is a holistic approach to determine the extent of Credit Risk for each borrower.
Credit Risk Assessment in Mortgage with its distinct subject matter has not been considered in this Point of View.
CURRENT SCENARIO
For the purpose of this Point of View the scope of Credit Risk is Transaction risk or Default risk. Default risk is defined as the risk of loss when the bank considers that the obligor is unlikely to pay its credit obligations in full or is more than 90 days past due.
Delinquencies are projected to rise slightly in the US as more aggressive lending practices return to the industry. Credit Risk Assessment has increased in significance in the light of the growing defaults.
Delinquencies in US Auto Loan market are forecasted to rise slightly in the 2013–2015 timeframe as more aggressive lending practices return to the industry.
Credit Risk Assessment using Modeling
Using models and algorithms rather than human judgment is prevalent in the high volume consumer lending business. Such models and algorithms are based on factual data, e.g. data collected by credit bureau agencies. A numerical score is often the result of a model that demonstrates the creditworthiness of borrowers. The data used for analysis and scoring spans across the individual account as well as the complete spectrum of accounts held by the customer. The data indicates the customer’s past behavior with respect to the repayment.
Consumer-credit risk analytics are typically strong computational techniques for finding patterns among extremely large datasets. However purely model based or statistical methods may not provide comprehensive credit assessment and subjective (non-statistical) credit assessment is also being introduced in businesses such as Auto loans.
Credit Risk Models
There are two main approaches to credit scoring – rules based scoring and statistical methods. Rules based scoring is scoring model based on the judgment of the individuals who develop it. It provides tool as an alternative to traditional ways. In rules based scoring, the credit
appraiser has to assign ratings to key factors that are critical to making the credit decision. It brings in consistency while evaluating different loan accounts.
Statistical methods focus on all variables that can indicate default using regression techniques. Statistical methods rank the variables based on their impact on the outcome. Some of the most commonly used methods include tree classification models, logistic or probit regression, neural networks, and duration models. The machine-learning algorithms include radial basis functions, tree based classifiers, and support-vector machines. {Please refer to Appendix for details on the various Statistical methods}
Model Development
During model development discriminant analysis is conducted on the historical data to predict the classification of future loans. Approval and scoring for individual loan applicants is based on their characteristics. The models also indicate probability of default for each customer. A bank can change its cut-off scores based on business strategy at that point in time. The threshold score could be used to determine level of approvals, or to limit delinquencies to an acceptable level. The threshold score is also one at which the lowest cost and lowest risk for a loan account is expected.
Some of the key aspects for developing a model include –
Sample Models
1. VantageScore®
{Reference – www.transunion.com/docs/rev/business/…/FS_ModelsOverview.pdf} VantageScore is a credit scoring model developed by the three of major US credit reporting companies- Experian, TransUnion and Equifax since 2006. It gives banks a way to deduce consistently the customer credit information across the three credit reporting companies. The credit information along with typical aspects leveling helps identify borrowers that are probable to be delinquent by 90 or more days within 24-month duration. All credit scores are measured in a numeric range, from 501 to 990. Factors influencing the VantageScore Credit Score are depicted below.
2. FICO Score
{Reference www.FICO.com }
FICO (Fair Isaac Corporation) Scoring solutions enables lenders with analytics with which the decision making processes are enhanced. In the US consumer credit risk domain, FICO Score is considered to be the standard scoring metric. Originally launched in the US, FICO scores are now available in 21 countries. An individual`s FICO score will range between 300 and 850. FICO 8, the latest US version, enhances the accuracy of predictability by up to 15%, especially for borrowers who have recently sought new credit and those with bad hits on credit history.
Credit Risk Assessment using Subjective Analysis
There have been increasing instances of prime borrowers turning into non-prime borrowers with downgrades after the credit was approved. This has posed challenges to the existing credit risk assessment methods. This necessitates focus on credit risk assessment of non-prime borrowers. Hence there is a need for subjective analysis to be included in credit risk assessment. This will help companies determine the non-prime borrowers who can meet the risk parameters as per policy. By including variables that are normally outside of traditional underwriting lenders can discover missing data or satisfy queries from the borrower’s credit history. To seek such subjective information, credit appraisers conduct interviews with prospective borrowers to understand the details behind missed payments. This changes the focus on the cause of the bad credit and not just the fact.
The success of this subjective analysis depends on the sincerity of the borrower in providing background information as well as the credit appraiser’s sound judgment. Based on the subjective assessment, the credit appraiser can determine whether the borrower has past the troubled credit period and can now be considered for new credit. Further analysis also needs to be done on whether the borrower can pay in the future considering his employment and income stability. During subjective analysis, lenders must use policies, procedures, and controls to comply with Regulation B-Equal Credit Opportunity. As an example of subjective factor, an auto insurance history of maintaining verifiable full-coverage will help make a strong case for the applicant borrower indicating his willingness to satisfy a contractual obligation.
Credit Risk Assessment for Auto, Consumer Durables and Personal Loans Across areas of consumer lending such as Auto, Consumer Durables and Personal Loans, the risk assessment approach has its own subtleties.
Credit Risk Assessment in Auto Loans
Having a predictive risk model for an auto loan company will help better predict the default probability for a borrower on the loan. With the use of the model, the auto loan company can make better decisions of approval or rejection of the auto loan application. This in turn helps establish standards thereby which maximum proportion of good borrowers is approved. While the model brings in consistency and objectivity via scoring, it is also recommended to have flexibility on scores and have a case-by-case basis evaluation to identify non-prime borrowers who may also qualify.
Some of the important factors in the auto credit risk assessment are –
* Loan-to-value (LTV) ratios
* Credit Bureau Score for the borrower
* Condition and Market value of the vehicle
* Age of vehicle taken as collateral
Credit Risk Assessment in Consumer Durables Loans
Consumer Durables Loans are peculiar due to small ticket size of loans and high volumes. They also demand quick turnaround for credit decision making. In the consumer durable loans business there is use of analytics (score card system) for credit risk assessment of borrowers. Typically processes that are automated are employed to provide fast response to borrowers. This fast turnaround time is possible due to interface with the Credit Bureaus who have vast repositories of data, ex. Credit Information Bureau (India) Limited (CIBIL), and in US- TransUnion, Experian & Equifax.
Credit Risk Assessment in Personal Loans / Line of Credit
Typically for a personal loan, borrowers have to undergo underwriting phases that include internal fraud and duplication checks, identity verification and external credit checks. The final outcome is a score, which determines approval or rejection. The funding/origination of a loan are based on verbal verification process that also includes contacting the customer directly. Risk assessment model for personal loans involves –
1. Creating a regression type credit scoring model that predicts overall customer risk 2. Identifying the predictors, and the drivers of being good borrowers and bad borrowers 3. Developing a classification for borrowers in terms of various levels of risk Risk scoring provides the level of credit risk for a customer. It may not provide an applicant’s individual assessment but provides statistical odds, or probability, that a borrower with any given score will be “good” or “bad”. Both traditional and non-traditional scorecards are used as tools for better decision making. Traditional scorecard includes attributes that are significant to differentiate good and bad borrowers. The final score of applicant is derived from the sum of individual score of each attribute contained in the applicant’s scorecard. To counter the constraint of inaccuracy in traditional scorecards, non-traditional ones include data mining such that it decreases the rate of wrong categorization of borrowers into good and bad. However, arriving at an accurate and optimal model is a result of iterations and expertise in data mining.
Existing Products in Credit Risk Assessment / Management
1. Moody’s Credit Risk Management –
It caters comprehensively to modeling of standard credit risk and scenario analysis. It addresses compliance to Basel II regulatory requirements. It the only key product that considers both economic as well as non-economic factors to account for variations in credit quality.
2. Moody’s and Equifax’s CreditForecast.com –
Developed by Moody’s and Equifax, this product provides a complete range of US Consumer credit services that includes advance insight into consumer’s balance sheets, data, analysis and predictions. This is available for a large variety of consumer credit, demographic and economic variables across geographic levels.
CHALLENGES IN CREDIT RISK ASSESSMENT AND MANAGEMENT
Model development for credit scoring and risk models is a perennial issue that lenders face. Refinement and calibration of models is also a challenge on a regular basis. Further impact on models happens with an intensive regulatory regime.
Some of the issues that model development faces are
* Constant change in regulation
* Lack of unified customer data that provides insights into the customer’s data across the organization * Lack of good underlying data. Data problems remain a nagging problem facing the lending industry. Accuracy and consistency is often missing in data quality. This also prevents the lender organization in meeting norms like Basel accord. * Models have lacked accuracy for Basel risk parameters [Probability of default (PD), Loss Given Default (LGD) and Exposure at Default (EAD). This increases the credit risk across the portfolio. FUTURE OF CREDIT RISK ASSESSMENT AND MANAGEMENT
Regulation has been a driving force for determining credit risk assessment at banks. Major regulatory policies such as Dodd – Frank reform, Consumer Protection Act and Basel III since the economic debacle of 2008 and others announced by the impacted nations of US, UK and Eurozone are pushing banks to be prepared and compliant. Increase in stress testing for credit exposure based on Basel III’s recommendations and thereby enable decision making.
Following are some of the ways in which banks are starting to better manage credit risk –
* High-end process management techniques like Lean and Six Sigma are being adopted more and more. While Six Sigma deals with decreasing variation from the value-added steps, Lean focuses on removing non-value added steps in a process. * Increasing involvement of senior management through risk goal setting, regular monitoring and collective responsibility. * Empowering the Credit Risk Officers and management heads for control and supervision, and to have deeper participation in implementation of polices. * Enhanced scoring analytics and credit reporting.
* Use of Information Technology – Increasing trend of big data management solutions, integrated risk management and sufficient IT infrastructure. BEST PRACTICES IN CREDIT RISK ASSESSMENT AND MANAGEMENT
Effective credit risk assessment and management would consist of well-defined business processes and policies, high-end analytics along with strong technology component. Some of the best practices in credit risk assessment that can help banks maintain a high credit quality are: * Instituting a suitable credit risk environment.
* Continuous monitoring of the portfolio quality.
* Leveraging Technology thereby removing manual intervention and bringing in data transparency. * Tight integration between compliance department (monitoring regulatory changes and impact) and the credit assessment department. * Stress testing, in-time and accurate risk computations, transparent credit risk as well as efficient credit risk reporting are some of the other component of effective credit risk management.
CONCLUSION
In summary, within the domain of consumer credit risk assessment, the statistical models of credit risk scoring provide greater accuracy and also enhance the efficiency of the risk scoring process. These statistical models should be used in tandem with the lender’s in-house models developed from internal analytics derived from the historical borrower data.
Multiple products are available in the market addressing credit scoring as well as assessment. These should be leveraged and integrated into the credit appraisal process of the lender.
Also, in addition to the risk scoring models, in the present economic scenario of increased population of sub-prime borrowers, subjective analysis with a human element has gained significance. This will help the lender to expand into the sub-prime market with an appropriate risk appetite.
The credit risk assessment as a function should be closely integrated with other departments of lending organizations such as Risk and Compliance. This has become more of a necessity for sound credit practice with the ever increasing influence of changes in the regulatory environment.
Appendix
Terminology:
1. Probability of Default (PD): It is the likelihood that a borrower will not adhere to its scheduled payment obligations. It impacts the rate of interest charged by the lender. It can be calculated for single borrower of a collective for set of borrowers.
2. Loss Given Default (LGD): It is the amount of credit loss that the lender gets exposed to, if its borrower does not satisfy its credit obligations.
3. Exposure at Default (EAD): It is the overall amount of lender’s credit risk exposure, exactly at the time of default. This is factor in calculation of economic and regulatory capital needs.
Statistical Methods:
1. Tree classification models: These are used to analyze and forecast the default probability for borrowers. CHAID – Chi-squared Automatic Interaction Detection – is a popular tree model which involves grouping of significantly similar independent parameters identified through regression methods.
2. Logistic regression model: It involves identifying independent parameters that are binary in nature, called Bernoulli variables, representing the two sides of default. It is typically used in multivariate data scenario with binary responses like the estimating credit risk through scoring. Since it allows predictions, it is typically used to forecast default over twelve month duration.
3. Probit regression Model: It is an alternative to Logistic regression. Herein, the dependent parameter is binary and estimation is via standard minimum probability method.
4. Neural network model: It includes program logics that get fine tunes automatically as more information becomes available. Due to this dynamic nature, it is beneficial in case of changes of business cycles.
5. Duration model: It is special time series model involving discrete state for continuous duration for random variables whose distribution over time is studied.
6. Radial basis functions methods: They provide approximation for multivariate functions for the case of data available in N dimensions that includes data sites and function values.
7. Support-vector machine model: It includes a method to identify the most significant features and thus facilitate stress testing of the forecasts. It benefit is lowered complexity of the model and the expected error rate.
8. Discriminate analysis: In involves classifying loan applicants into high-low risk of default categories, instead of calculating the probability of default.
References:
http://files.ots.treas.gov/422034.pdf
http://files.ots.treas.gov/422059.pdf
http://rbidocs.rbi.org.in/rdocs/notification/PDFs/9492.pdf
http://fic.wharton.upenn.edu/fic/papers/02/0209.pdf
http://www.virginia.edu – Link
http://www.occ.gov/publications/publications-by-type/comptrollers-handbook/lpm.pdf Office of Thrift Supervision January 2000 Examination Handbook 217.9 http://www.sas.com/ -Link1 ; Link2
http://www.ey.com/ – Link1; Link2
http://www.mckinsey.com/ – Link
http://pages.stern.nyu.edu/~ealtman/2-%20CopManagingCreditRisk.pdf http://www.bisolutions.us – Link
http://www.sovcredit.co.uk/blog/?p=72
http://www.sungard.com/pressreleases/2011/adaptiv063011.aspx http://towergroupedge.exbdblogs.com/2012/03/13/us-consumer-credit-scoring-and-lending-market-forecast http://www.philadelphiafed.org/ -Link
http://business.myjoyonline.com/pages/finance/201112/78058.php http://www.kpmg.com.au/Portals/0/eiu_Risk_Management.pdf
http://www.bis.org/publ/bcbs75.htm
http://www.businesswire.com – Link
http://www.pwc.com/us/en/consumer-finance/publications/assets/cfuwinter05.pdf http://www.economy.com/home/products/consumer-credit-analytics.asp?src=left-nav http://www.finextra.com/ – Link
http://www.tradeinvestafrica.com/feature_articles/1117760.htm
PD / LGD /EAD
http://fic.wharton.upenn.edu/fic/papers/04/0401.pdf
www.bankopedia.net
http://lexicon.ft.com/Term?term=probability-of-default
CHAID
http://www.hanken.fi – Link
Logistic
http://bib.irb.hr/datoteka/466476.sarlija_soric_vlah_vojvodic.pdf http://upetd.up.ac.za/thesis/available/etd-08172010-202405/unrestricted/dissertation.pdf Probit
http://www3.iam.metu.edu.tr/iam/images/2/21/%C3%96zgesezginthesis.pdf Radial basis functions
http://catdir.loc.gov/catdir/samples/cam033/2002034983.pdf
http://www.cin.ufpe.br/~tbl/artigos/applied-intelligence2005.pdf Duration Model –
www.diva-portal.org/smash/get/diva2:221741/FULLTEXT01
Support Vector Machine – Link